Temporal seizure propagation patterns in epilepsy
A probabilistic model as a potential tool to aid clinicians in identifying the seizure focus
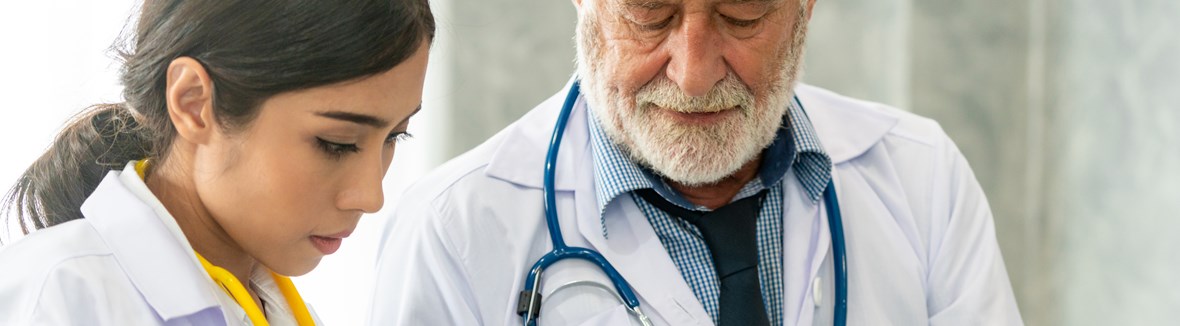
Drug-resistant epilepsy is a class of epilepsy where medication fails to control seizures and is observed in nearly 25% of epilepsy patients. In these cases, clinical treatment usually involves surgical resection of brain regions that are considered to be originating seizures. Success rates of epilepsy surgery, ranging between 50% and 60%, dictate the need to develop better methods to identify an epileptogenic zone (EZ). Focal epileptic seizures are characterized by seizures originating in one or more regions, generally referred to as EZ, and propagating to other regions that are connected to regions in the EZ, generally referred to as a propagation zone (PZ). These authors propose a Bayesian framework based on a dynamical model of epileptic seizures, namely Epileptor, to identify spatiotemporal seizure propagation patterns.
A Bayesian inference paradigm
Herein, a Bayesian inference paradigm was used to perform model inversion. Bayesian inference offers a flexible framework to incorporate any prior knowledge such as plausible range of model parameters, dynamics of unobserved brain states, and prior hypothesis on the seizure focus. This information constrains the parameter space, thus enabling efficient exploration of posterior distribution of parameters.
In the field of neuroscience, Bayesian inference has been extensively and successfully used for model inversion by a class of models called dynamic causal modeling (DCM). DCM is a Bayesian framework to infer physiological mechanisms that can generate observations obtained from various neuroimaging techniques such as functional magnetic resonance imaging (MRI) and electroencephalography (EEG).
A workflow to identify seizure propagation patterns
The workflow for identifying seizure propagation pattern used consists of the following steps: (a) estimating structural connectome (SC) and source to sensor space transformation from diffusion MRI data and electrode implantation, respectively; (b) extracting data features, log power over a sliding window, from the observed SEEG data; (c) defining a generative model that describes the joint probability density over the observations and Epileptor model parameters; (d) performing model inversion using MAP techniques in order to infer the model parameters and latent source states that best fit to the observed SEEG log power; (e) computing the seizure-onset times of all regions recruited by the seizure by thresholding the inferred latent states; and (f) identifying the EZ and PZ: all regions with onset times between the lowest onset time and a small onset tolerance window are classified as part of EZ, and regions with onset times greater than this window are classified as part of PZ.
The proposed probabilistic model is a potentially valuable tool to aid clinicians in identifying the seizure focus.
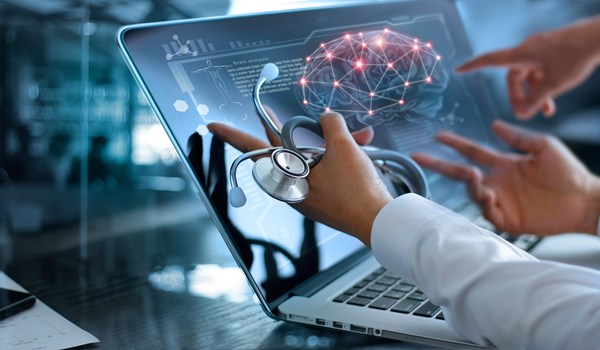
Testing the Bayesian framework
The authors tested the model against a retrospective patient cohort of 25 drug-resistant epilepsy patients who underwent surgery. The cohort was divided into two groups based on the outcome the of surgery as follows: (i) Engel score I and II: patients who are either seizure-free or show rare disabling seizures and (ii) Engel score III and IV: patients with minimal or no worthwhile improvement. Out of the five brain regions in the clinical EZ hypothesis, the model predicted four regions to be recruited by the seizure with one subcortical region (right hippocampus) in EZ and three regions (right thalamus proper, right amygdala, and ctx-rh-G-oc-temp-med-Parahip) as part of PZ. In the patients who were seizure free after surgery, model predictions showed a good match with the clinical hypothesis. In patients where surgery failed to achieve seizure freedom, the model predictions showed a strong mismatch.
Implications for daily practice
By combining a priori knowledge of the seizure dynamics, anatomical connections, and clinical expertise with observed intracranial recordings in a Bayesian framework, these authors demonstrated that whole-brain network models can be inverted to build individualized in silico models of a patient’s seizure dynamics. The strategy to construct a personalized whole-brain model allows the possibility to refine clinical hypotheses and explore novel therapeutic techniques to improve outcomes of surgery for epilepsy. In addition to being a potentially valuable tool to aid the clinicians in identifying the seizure focus, the proposed probabilistic model could also be used to investigate reparameterizations of the generative model to sample the posterior efficiently using Markov chain Monte Carlo techniques.
- Vattikonda AN, Hashemi M, Sip V, et al. Identifying spatio-temporal seizure propagation patterns in epilepsy using Bayesian inference. Commun Biol. 2021;4(1):1244.
NO13967P, Oktober 2022